Table Of Content
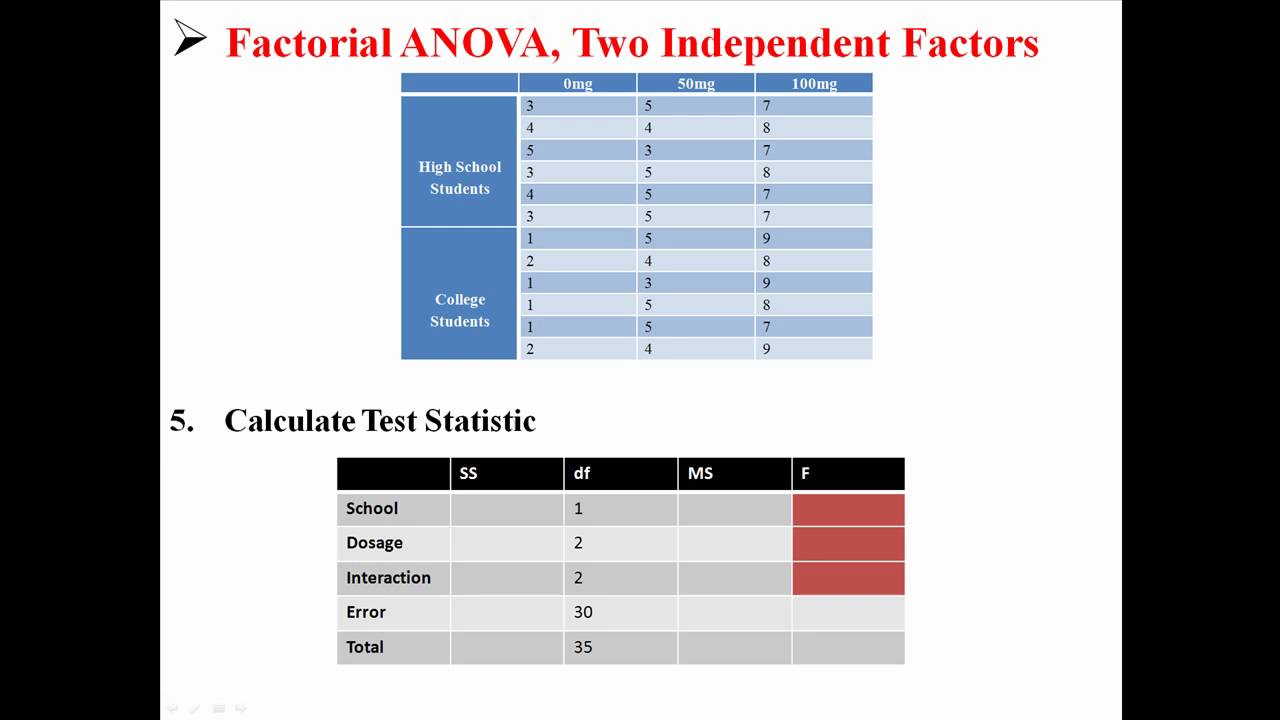
However, it is important to make sure the individual dependent variables are correlated with each other by computing an internal consistency measure such as Cronbach’s \(\alpha\). If they are not correlated with each other, then it does not make sense to combine them into a measure of a single construct. If they have poor internal consistency, then they should be treated as separate dependent variables. This example nicely illustrates the distinction we made between simultaneity and dependency. On the two aspects of the timing dimension, this study was a concurrent-dependent design answering a set of related research questions.
3. Factorial designs: Round 2¶
Introverts perform better than extraverts when they have not ingested any caffeine. But extraverts perform better than introverts when they have ingested 4 mg of caffeine per kilogram of body weight. We agree with Greene (2007), who states that the value of the typological approach mainly lies in the different dimensions of mixed methods that result from its classifications. In this article, the primary dimensions include purpose, theoretical drive, timing, point of integration, typological vs. interactive approaches, planned vs. emergent designs, and complexity (also see secondary dimensions in Table 1). Unfortunately, all of these dimensions are not reflected in any single design typology reviewed here. Contrary to other authors, however, we do not consider these designs as a feature of a whole study, but rather, in line with Guest (2013), as a feature of one part of a design in which one qualitative and one quantitative component are combined.
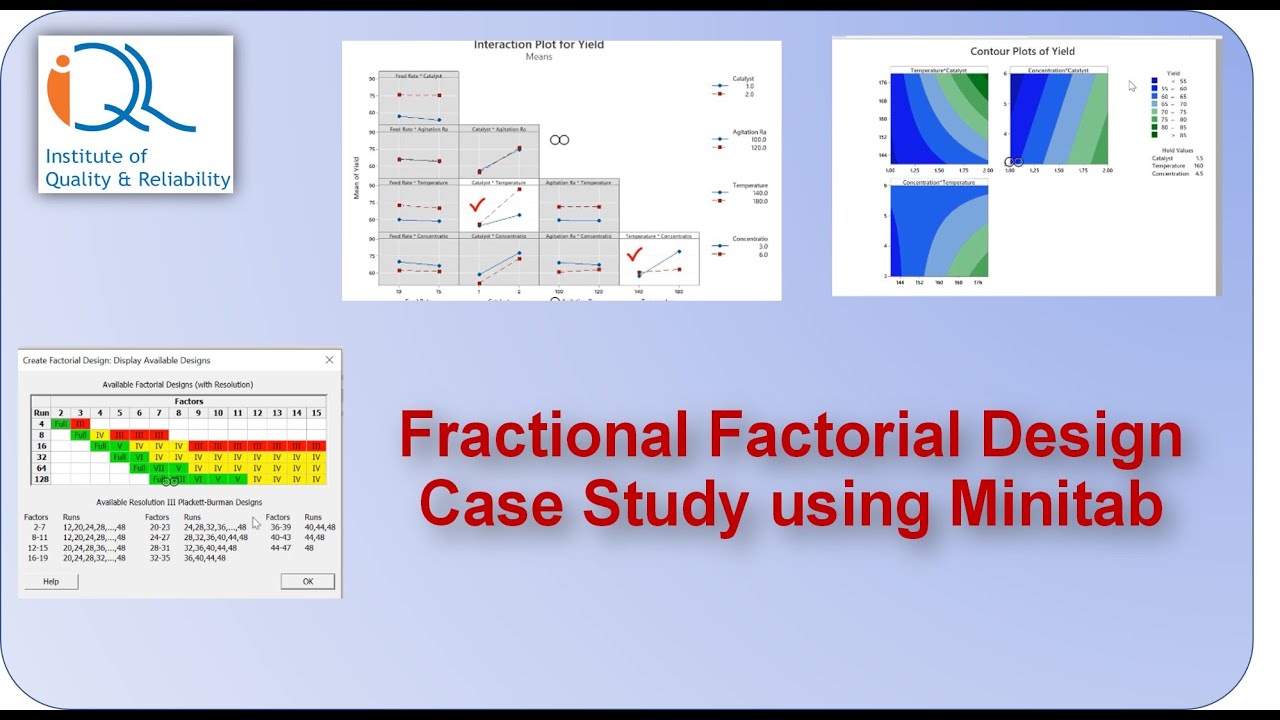
1.2. Measures of Different Constructs¶
Some authors state that design typologies are particularly useful for beginning researchers and interactive approaches are suited for experienced researchers (Creswell and Plano Clark 2011). This makes an interactive approach desirable, also for beginning researchers. Substantively, it can be useful to think of integration or mixing as comparing and bringing together two (or more) components on the basis of one or more of the purposes set out in the first section of this article.
2. Multiple Independent Variables¶
For this mixed-factorial design, we need to account for the fact thatwe have multiple observations coming from each person, so we will add arandom-effect of “subID”. After accounting for this statisticaldependence in our data, we can now fairly test the effects of Age Group,and Condition with residuals that are independent of each other. Again, for our purposes, the critical thing to note is that theF-values for the main-effects and interactions are the same between ourRM ANOVAs and the mixed-effects model. Without delving into themathematical details, this is a good demonstration that the appropriaterandom-effects our regression model make it analogous to the factorialANOVA. This allows us to capitalize on the benefits of mixed-effectsregression for designs that we would normally analyze using factorialANOVA.
A mixed methods design can be thought out in advance, but can also arise during the course of the conduct of the study; the latter is called an “emergent” design (Creswell and Plano Clark 2011). Emergent designs arise, for example, when the researcher discovers during the study that one of the components is inadequate (Morse and Niehaus 2009). Addition of a component of the other type can sometimes remedy such an inadequacy. Initiation, for example, is the further exploration of unexpected outcomes.
3.7. Interactions¶
For example, all participants could be tested either while using a cell phone or while not using a cell phone and either during the day or during the night. In a within-subjects factorial design, all of the independent variables are manipulated within subjects. All participants could be tested both while using a cell phone and while not using a cell phone and both during the day and during the night. The advantages and disadvantages of these two approaches are the same as those discussed in Chapter 6. The within-subjects design is more efficient for the researcher and controls extraneous participant variables.
These mixed methods researchers are likely to believe that qualitative and quantitative data and approaches will add insights as one considers most, if not all, research questions. The key point of this section is for the researcher to begin a study with at least one research question and then carefully consider what the purposes for mixing are. One can use mixed methods to examine different aspects of a single research question, or one can use separate but related qualitative and quantitative research questions. In all cases, the mixing of methods, methodologies, and/or paradigms will help answer the research questions and make improvements over a more basic study design. Fuller and richer information will be obtained in the mixed methods study. Figure 8.5 shows examples of these same kinds of interactions when one of the independent variables is quantitative and the results are plotted in a line graph.
Application of response surface methodology for COD and ammonia removal from municipal wastewater treatment ... - ScienceDirect.com
Application of response surface methodology for COD and ammonia removal from municipal wastewater treatment ....
Posted: Thu, 07 Jul 2022 13:40:00 GMT [source]
Non-Experimental Studies With Factorial Designs
Another important use of complex correlational research is to explore possible causal relationships among variables. This might seem surprising given that “correlation does not imply causation”. It is true that correlational research cannot unambiguously establish that one variable causes another. Complex correlational research, however, can often be used to rule out other plausible interpretations. Importantly, the effect of the gas variable on driving depends on the levels of having a key. Or, to state it in reverse, the effect of the key variable on driving depends on the levesl of the gas variable.
Assuming the restricted version of the mixed effect model, Minitab’s balanced ANOVA routine output is given as follows. A nice collection of examples of mixed methods studies can be found in Hesse-Biber (2010), from which the following examples are taken. Factorial designs are the basis for another important principle besides blocking - examining several factors simultaneously. We will start by looking at just two factors and then generalize to more than two factors. Investigating multiple factors in the same design automatically gives us replication for each of the factors.
Similarly, the empirical outcome/effect and process in a study with the purpose of expansion might be investigated independently, or the process study might take the effect/outcome as given (dependent). The overall goal of mixed methods research, of combining qualitative and quantitative research components, is to expand and strengthen a study’s conclusions and, therefore, contribute to the published literature. In all studies, the use of mixed methods should contribute to answering one’s research questions.
But there are also plausible third variables that could explain this relationship. It could be, for example, that people who are lower in SES tend to be more religious and that it is their greater religiosity that causes them to be more generous. Or it could be that people who are lower in SES tend to come from certain ethnic groups that emphasize generosity more than other ethnic groups. The researchers dealt with these potential third variables, however, by measuring them and including them in their statistical analyses. They found that neither religiosity nor ethnicity was correlated with generosity and were therefore able to rule them out as third variables. This does not prove that SES causes greater generosity because there could still be other third variables that the researchers did not measure.
(c) Illustration – refers to the use of qualitative data to illustrate quantitative findings, often referred to as putting “meat on the bones” of “dry” quantitative findings. I have a Master of Science degree in Applied Statistics and I’ve worked on machine learning algorithms for professional businesses in both healthcare and retail. I’m passionate about statistics, machine learning, and data visualization and I created Statology to be a resource for both students and teachers alike.
Here we have shown that one often needs to construct a research design to fit one’s unique research situation and questions. This is why we emphasize in this article the importance of learning to build on simple designs and construct one’s own design for one’s research questions. This will often result in a combination or “hybrid” design that goes beyond basic designs found in typologies, and a methodology section that provides much more information than a design name. Notice that Morse and Niehaus (2009) included four mixed methods designs (the first four designs shown above) and four multimethod designs (the second set of four designs shown above) in their typology.
No comments:
Post a Comment